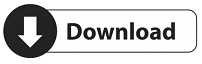
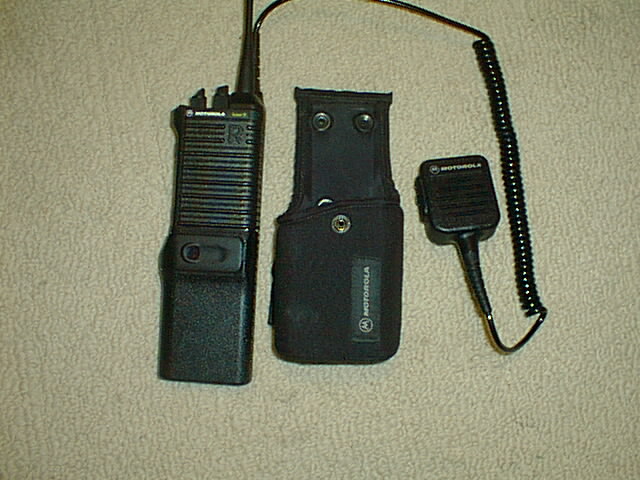
Stroke classification is important because many therapeutic decisions are dependent on its pathophysiology. Interpretation: ASTRO-X, a deep neural network developed to diagnose cardioembolic stroke based on chest radiographs, demonstrated good classification performance and biological plausibility. Compared with cases predicted as non-cardioembolism by ASTRO-X, cases predicted as cardioembolism by ASTRO-X had higher left atrial volume index and lower left ventricular ejection fraction in echocardiography. The gradient-weighted class activation map demonstrated that ASTRO-X was focused on the area where the left atrium was located. External testing was performed with 750 chest radiographs of patients with acute ischaemic stroke from 7 hospitals.įindings: The areas under the receiver operating characteristic curve of ASTRO-X were 0.86 (95% confidence interval, 0.83–0.89) and 0.82 (95% CI, 0.79–0.85) during the internal and multicentre external testing, respectively. Gradient-weighted class activation mapping was used to evaluate the region of focus of ASTRO-X. The performance of ASTRO-X was evaluated using the area under the receiver operating characteristic curve. A densely connected convolutional network (ASTRO-X) was trained to diagnose cardioembolic stroke based on chest radiographs.
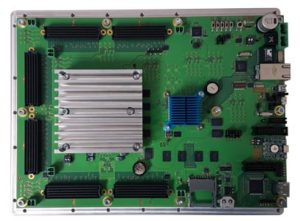
Chest radiographs were randomly partitioned into training/validation ( n = 3,255) and internal test ( n = 809) datasets in an 8:2 ratio. Methods: Overall, 4,064 chest radiographs of consecutive patients with acute ischaemic stroke were collected from a prospectively maintained stroke registry. This study aimed to develop a deep convolutional neural network that could diagnose cardioembolic stroke based on chest radiographs. Background: Although chest radiographs have not been utilised well for classifying stroke subtypes, they could provide a plethora of information on cardioembolic stroke.
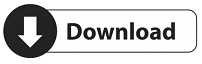